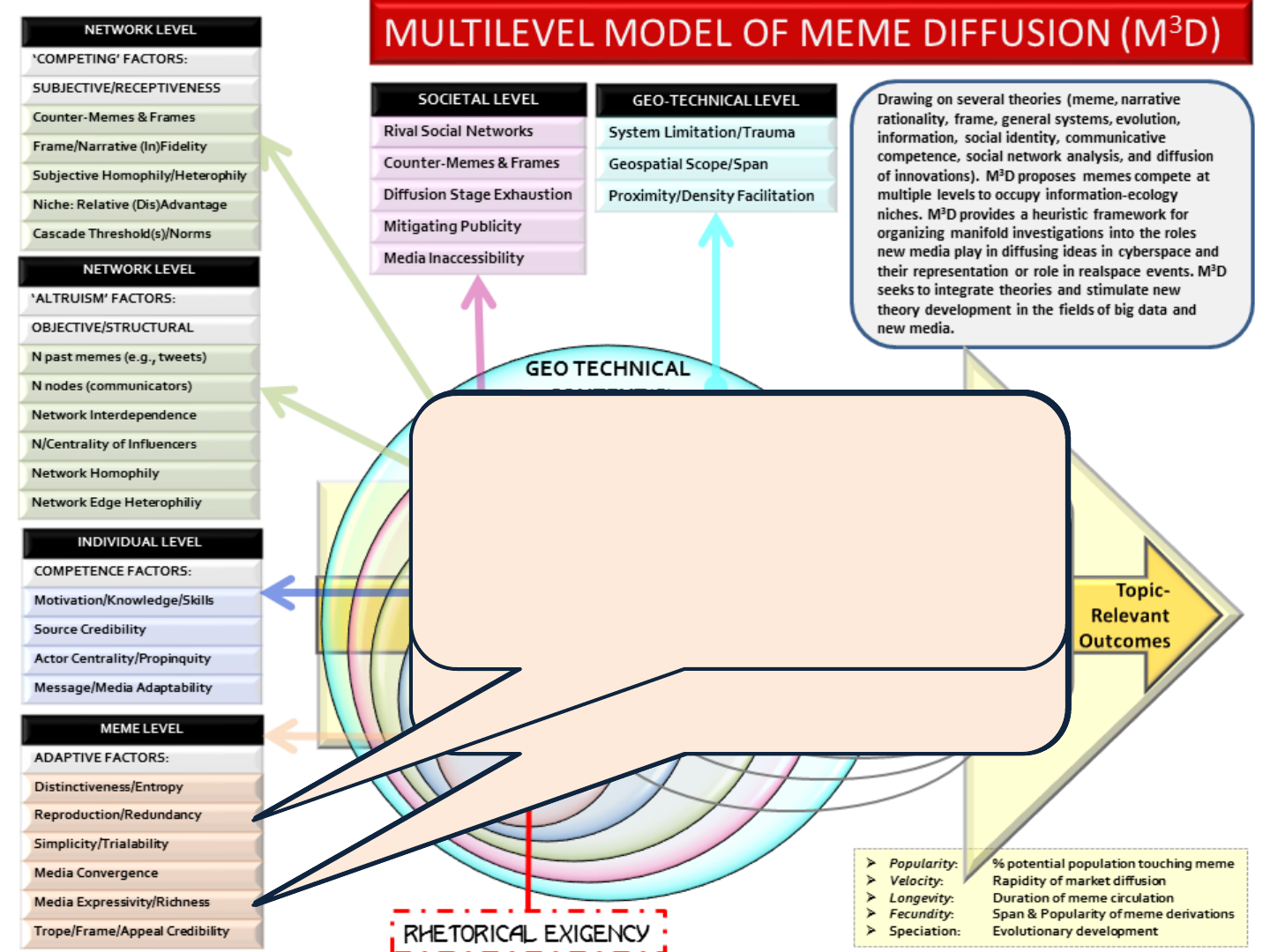
LINGUISTIC AGENCY:
• Linguistic agency of disease severity of
disease;
• Linguistic agency of vax belief in
mandatory vaccination policies (Bell et al.,
2014)
NARRATIVE FORM:
• Narratives > statistics (Betsch et al. 2011;
see also: Shelby & Ernst, 2013))
• Narratives + statistics + disclaimer (Betsch et
al., 2013)
NARRATIVE FORM:
“we observed a stable narrative bias…
normatively irrelevant information conveyed
by narratives from online discussion boards
systematically increased perceptions of
vaccination risks” (Bell et al., 2014)
GIST-STATISTIC-STORY:
Both providing a clear gist and providing
statistics increased Facebook sharing of
Disneyland vax articles on Facebook, but not
stories (Broniatowski et al., 2016)
APPEAL TYPE:
An experiment comparing 4 appeal types: (a)
lack of evidence of harm; (b) dangers of the
diseases; (c) images of saved children; & (d)
narrative of saved child, showed none
worked, and some backfired (Nyhan et al.,
2014; cf. MacDonald et al., 2013)
FEAR APPEALS:
Parental vax resistance is based more on fear
of actively doing harm (vaccinating) than not
doing something (not vaccinating) that results
in harm (Wroe et al., 2004)
SENTIMENT:
Mere exposure to negative vax sentiments in
tweets increases the likelihood of subsequent
posting of negative opinions on vaccines,
supporting the value of denialism as a
rhetorical strategy (Dunn et al., 2015)
REMINDERS:
Patient reminder messages demonstrated a
significant predictive role in increasing adult
patient immunization (OR = 2.52-3.80; Stone
et al., 2002; see also: Lau et al., 2012)
EFFECTS OF MEDIUM:
First ‘hit’ exposure to anti-vax websites
knowledge, fear of adverse effects,
whereas first hit pro-vax sites vax
knowledge (Allam et al., 2014)
EFFECTS OF MEDIUM:
97% of health info. seekers accessing web
stick with initial 10 hits (Eyesenbach, 2002);
anti-vax web info for > 10 min. = vax
exemption intention (Betsch et al., 2010)